You invested in big data technologies thinking it will help you understand your audience better. You switched to smart CRMs, hired data scientists to help you make sense of this data. You used more apps, procured more solutions to get more data. Yet, you’ve not been able to understand or get an accurate, unified, 360 view of your customer. You don’t have a customer personalization plan. Your marketing, sales, and customer service teams are all working on siloed data. Your organization is overwhelmed with data that’s just being stored in a data lake but has no use.
If this sounds familiar, you’re not alone.
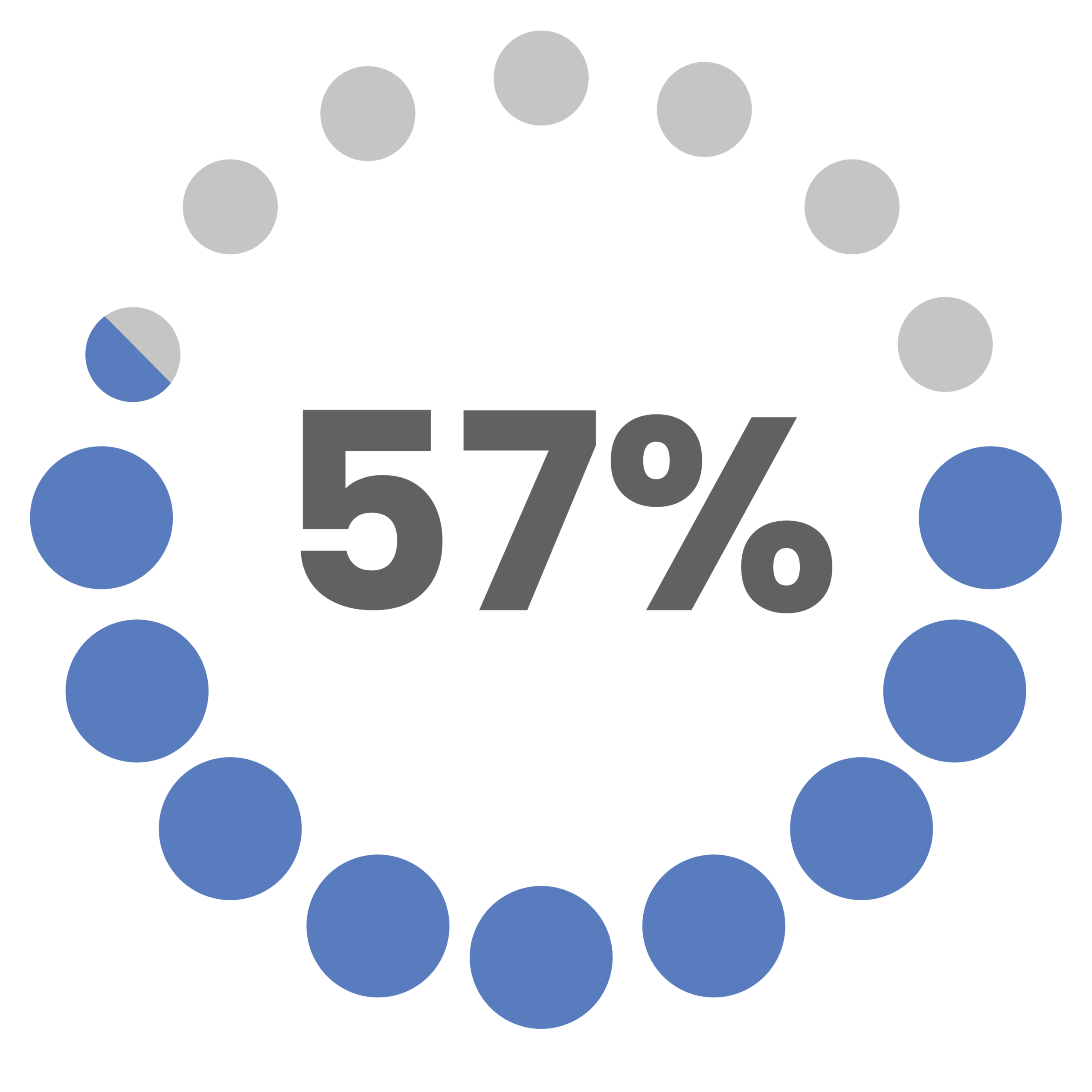
of companies in a research conducted by Marketing Week reported they have not yet built a 360 customer view.
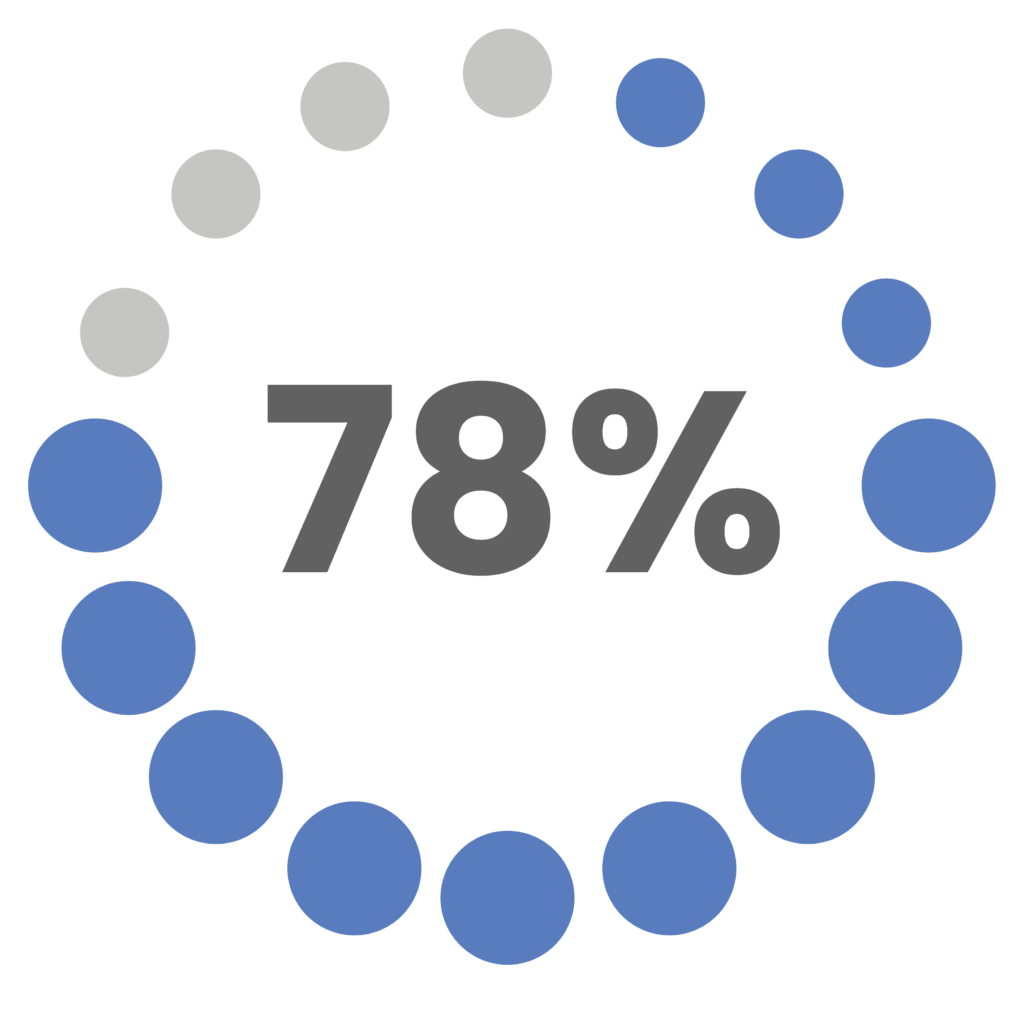
of companies say that the single customer view is extremely important to their organization. You need a 360-customer view. And this guide will show you how.
Why it’s important to consolidate your Data to get a 360 view?
A 360 customer view is a holistic, consolidated and consistent representation of an organization’s customer data. Customers expect interactions with an organization to reflect their current profile, history, and preferences. For this reason, this unified view is particularly important when organizations interact with customers through multiple channels.
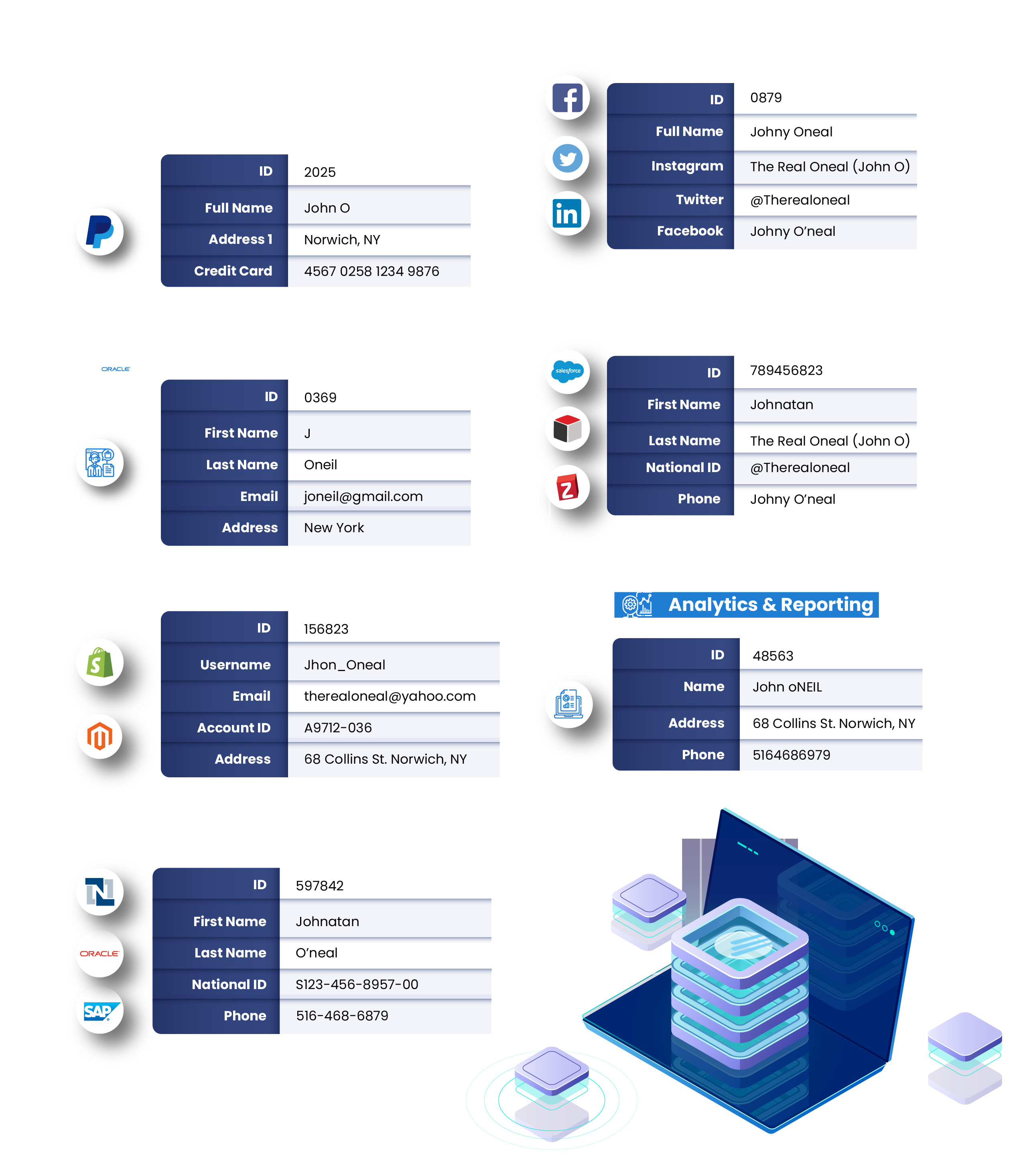
And when it comes to interactions through multiple channels, we invariably get multiple data sources. If an average enterprise uses Salesforce, HubSpot, Google, Oracle to manage their customer data, in addition to external third-party brokers or partners’ platforms, they are dealing with an overwhelming amount of data. All this data from internal and external sources need to be integrated to build this view.
But this is easier said than done.
Businesses do not have access to accurate data to get a 360 customer view. And that’s perhaps the biggest roadblock to making sense of customer data.
Single Customer View as the Most Pressing Data Quality Challenge
In our years of experience working with Fortune 500 companies, we can safely conclude that achieving a single customer view is a pressing challenge, made complicated by poor data quality.
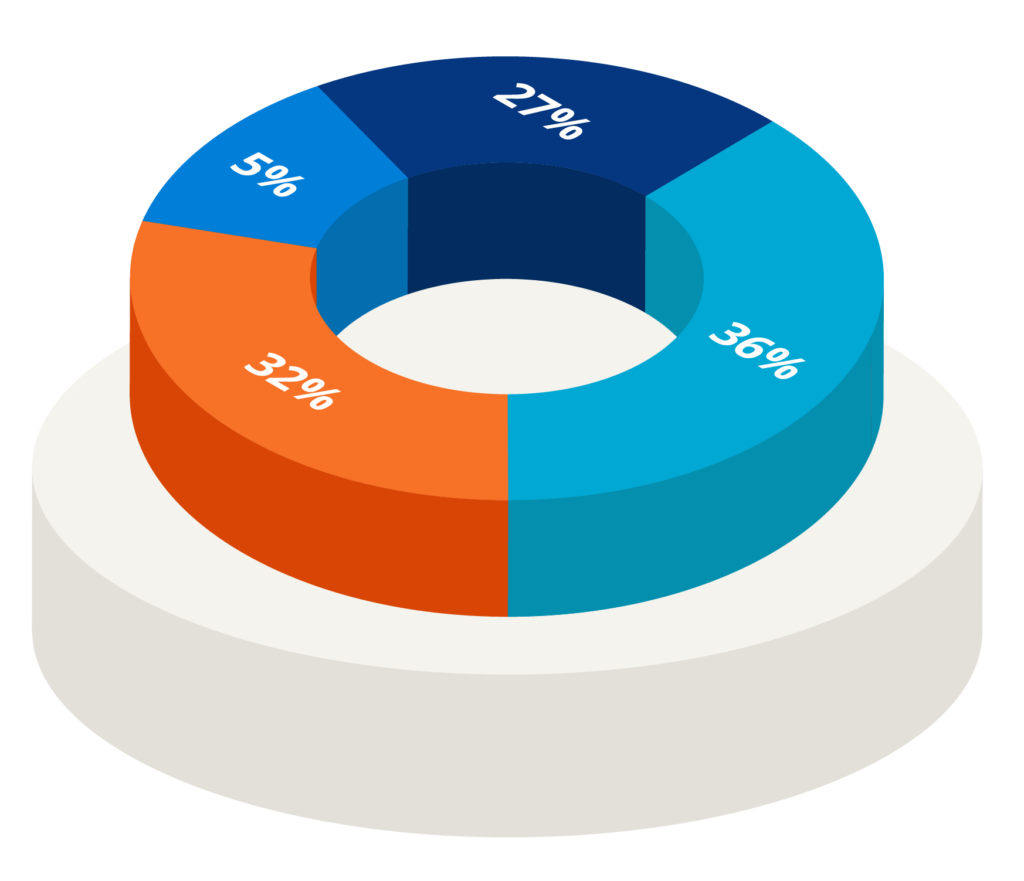
Single Customer View
Analytics & Reporting
Data Compliance
Real-Time Processing
There are a few key reasons as to why organizations are still struggling with data quality. Some of these could be attributed to:
Poor Data Management
While companies are spending millions in data lakes, cloud solutions, data warehouses and big data platforms, they fail to implement a strong data management strategy. Data is still being collected from disparate sources, dumped into data lakes. Trained data scientists are expected to spend 80% of their time cleaning this data and making it usable.
Lack of a Data Quality Strategy:
As of 2016, over 66% of companies did not have a data quality strategy in place. This figure still
holds. Companies don’t have a plan and don’t have the resources and the mindset to consider data quality as a significant challenge.
Poor Operational Efficiency:
Executive leadership is unaware of the problems with their data. Business users don’t have the right tools to fix issues. Customers are at the receiving end of bad data. The organization’s efficiency is under threat.
Data quality is not just a technical oversight. It’s a mindset that organizations have yet to align everyone to. While the executive leadership talks about mergers & acquisitions, clouds and data lakes, the employee using the data is nowhere aligned with a data-driven goal or mindset.
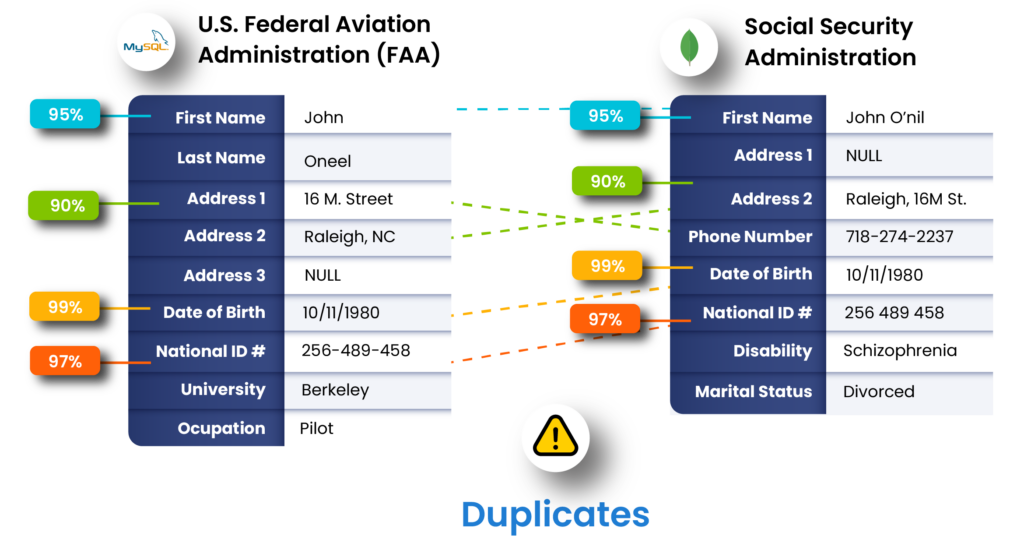
How Data Quality Affects Customer Data – A Case Study
Bell Bank, a renowned bank with branches in all 50 states of the US has a goal – to unify data from internal and external sources and get a singular customer view that can be used to create personalized services.
But before that’s possible, the bank has to deal with:
Disparate Data Sources: The bank’s external vendors and third-party services manage data using their platforms and solutions. When the bank wants to get the 360-customer view, it has to retrieve this data from third-party sources with its inconsistencies and variability.
In this specific example, disparate data is due to external associations, which makes sense. In most companies though, internal teams use varying apps and systems to collect and store data which makes it even more difficult to resolve. Even if the company uses one central platform, the lack of a definite process and adherence to data quality rules causes disparate information.
Duplicate Records: Duplication of data is the most dangerous data quality problem as it’s so difficult to identify. In the case of Bell Bank, the consolidation of customer data resulted in significant duplicates. It was bound to happen – when you consolidate data from multiple sources, chances are you’re storing multiple versions of a single entity’s records.
Take a look at the example below:
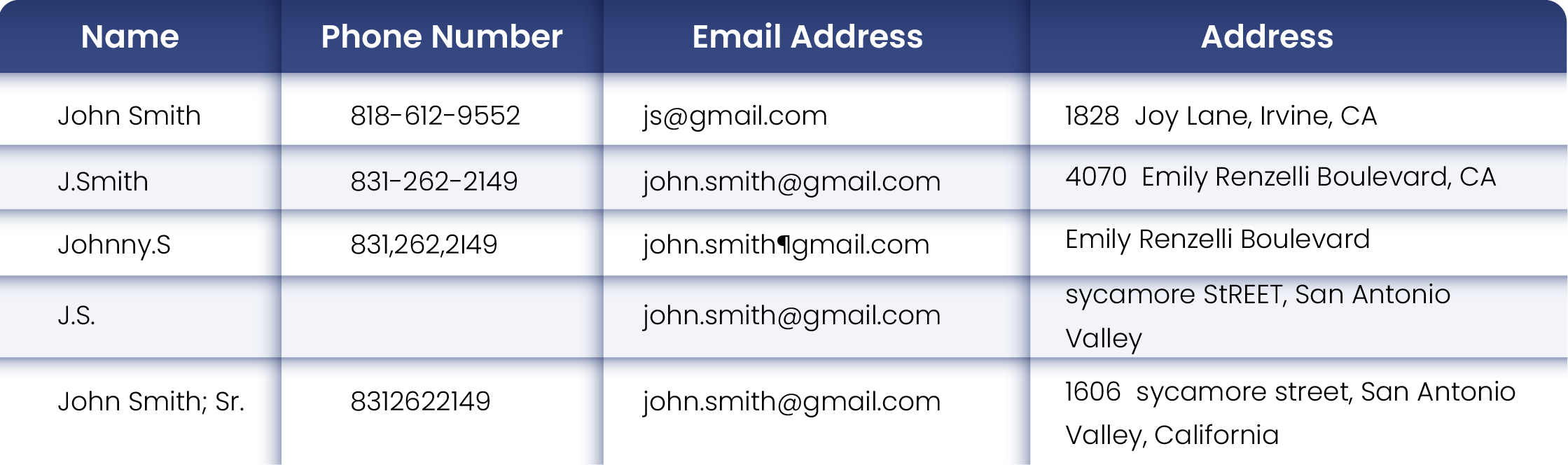
This is five different versions of one entity (individual) created by perhaps five different people, stored in different platforms. The entity has changed phone numbers, email addresses, and physical addresses in the five years that he has been with the bank. This kind of duplicate is the most difficult to weed out because none of the values are deterministic.
Messy Data: Not only is this data duplicated, it is also severely messy. There are no standards and no formatting rules are applied. Abbreviations, punctuations, misspelled names are degrading the quality and affecting the data’s usability. While this may seem like a small problem, companies end up spending millions in hiring data analysts just to identify these errors and clean them up. Moreover, this messy database can result in data security problems, embarrassing mistakes and annoyed customers.
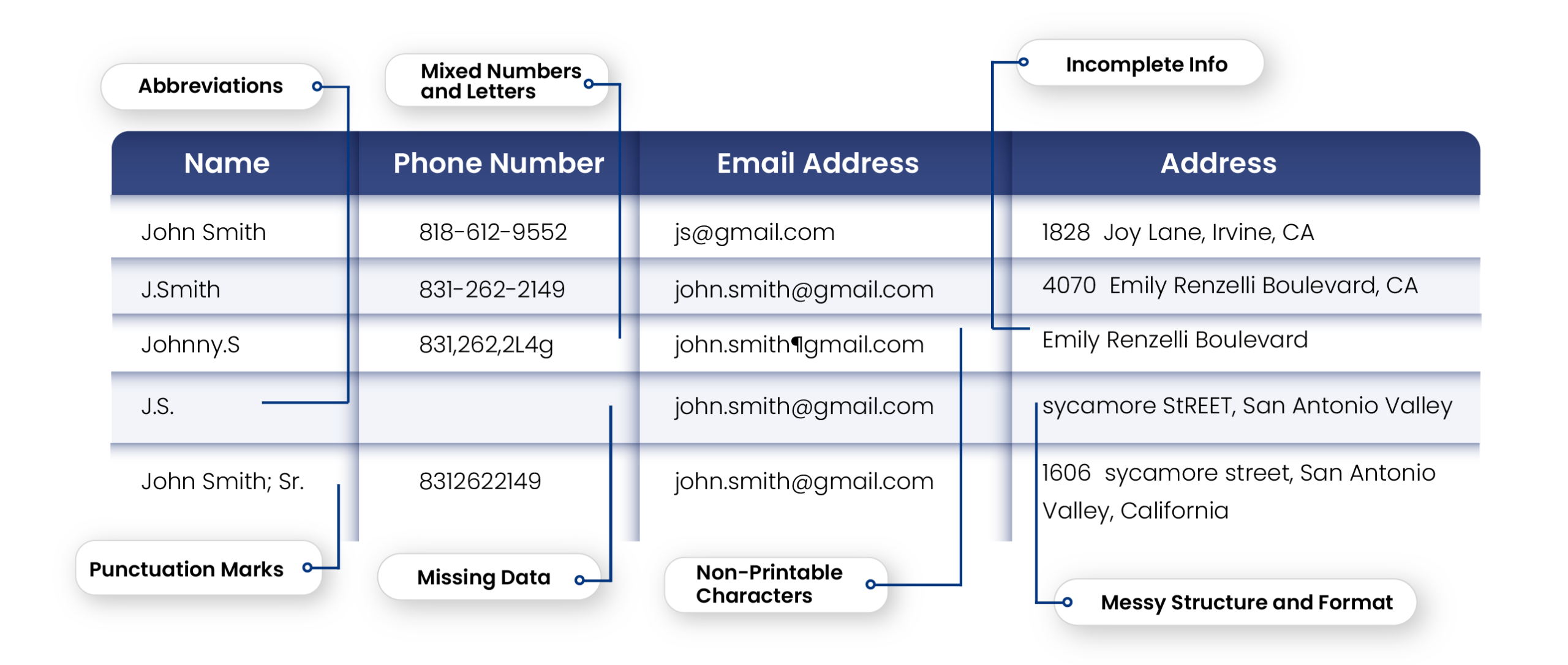
The starting point to a 360 customer view is the most basic – clean, dedupe & standardize your data before consolidating it.
Why Data Quality Matters?
You don’t have to wait for a high-level goal like customer personalization to maintain the records of your data. If you implement a strong data quality policy now, you’ll be saving up on unnecessary expenses & costly mistakes while improving operational efficiency. In fact, there are 101 important reasons as to why high-quality records matter. Here’s a quick list of the most common ones.
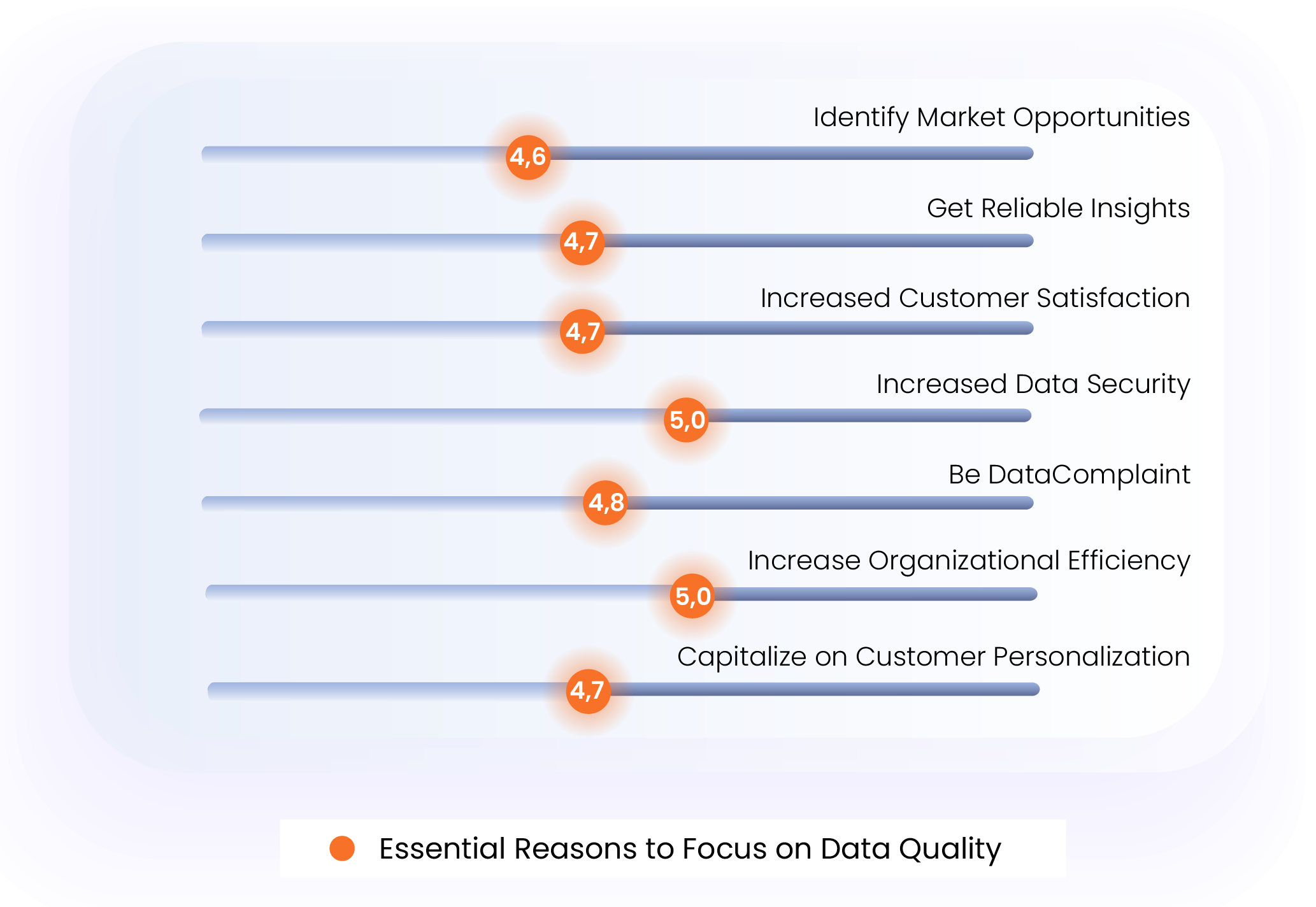
The Process – How Can Customers Get this View?
Now that you know the what and why; let’s discover the how.
We recommend following a ten-step framework which also forms the basis of Data Ladder’s data quality solution. Following a holistic data quality process, DataMatch Enterprise, Data Ladder’s flagship solution allows users to:
Integrate data from 150+ sources.
Profile millions of rows of data to discover underlying errors such as format issues, spelling mistakes, missing information, and overall column health.
Clean and standardize data according to pre-defined business rules.
Validate and standardize address data according to USPS and other government data bases.
Match and dedupe data.
Merge records.
Automate cleaning schedule.
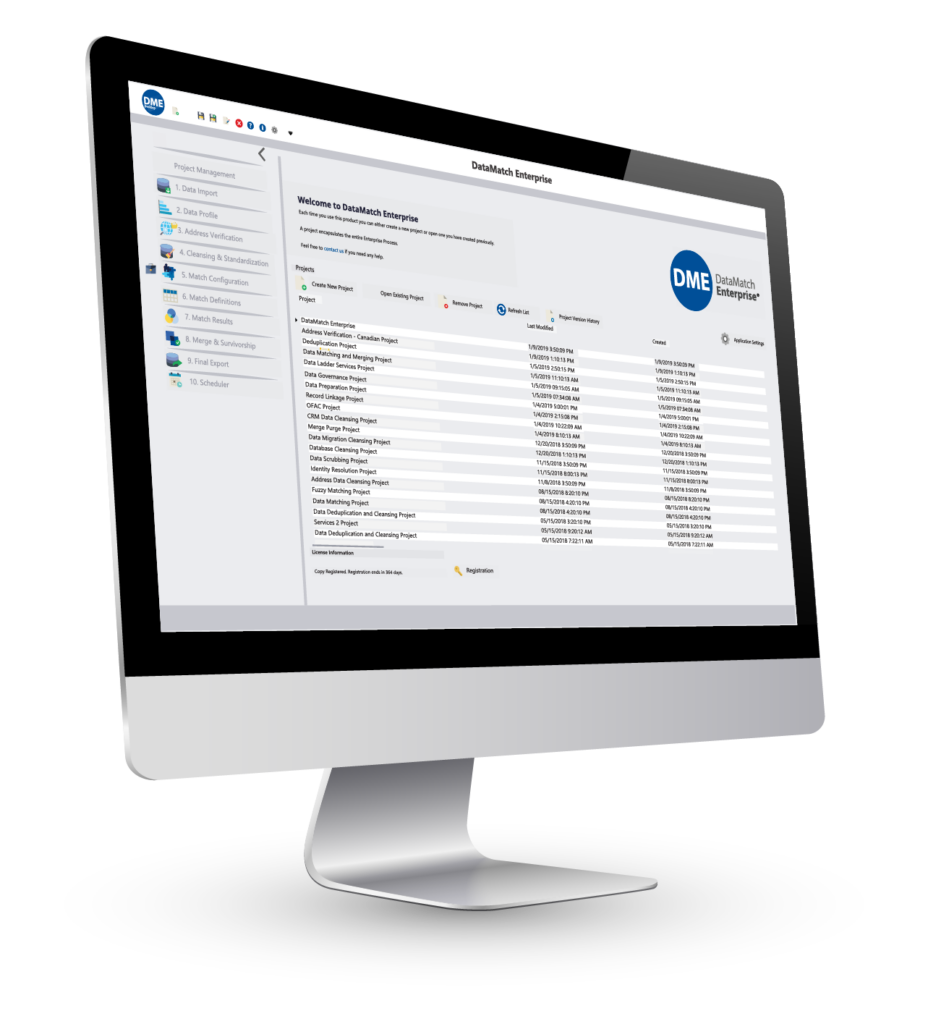
Customers who have implemented this framework were in a much better position to make sense of their data and were able to successfully get a single customer view to implement their customer personalization goals.
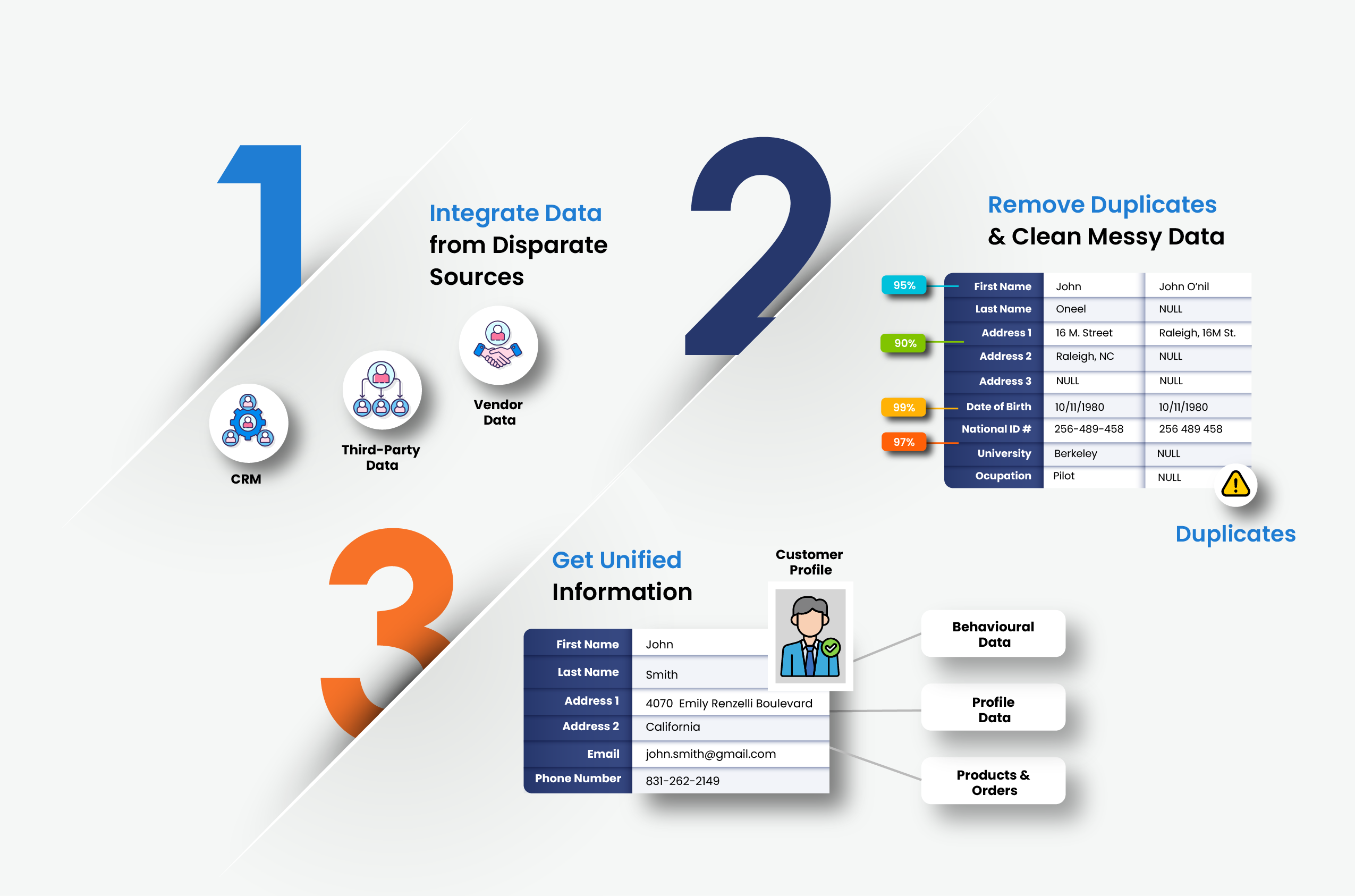
1. Decide Why You Want a 360 Customer View: You’ll need to brainstorm with your team here.
Try answering the following questions:
Why do we need a 360 customer view?
What are the outcomes we expect with this view?
Do we need a 360 customer view of ALL our customers?
Do we have the bandwidth, team and resources to manage the project?
Do we understand our data obstacles?
2. Create a Manageable Goal: Often, companies start big, only to fail soon. It’s recommended that
you start this project with smart and manageable goal. Say you want to launch a loyalty card service for your top 500 most loyal customers, categorized based on the number of years and the services they have availed. This seemingly small goal will take a significant amount of time as you sort through your data to retrieve the list of 500 customers. It’s here that the hurdles of duplicates and disparate data will pop up and cause delays.
3. Identify & Integrate Your Data Sources: Identify and integrate your data first-party, second-party, and third-party data sources that contain the customer information that you want to bring together.
Which format are you receiving data from your partners and brokers in?
Which applications or databases do you have in place internally?
Will you be connecting to public data sources, if so, which format will you download those list in?
Customer data that you’d need to build a true Single Customer View can include:
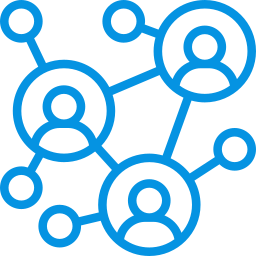
Social Media

Demographics
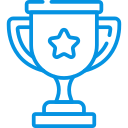
Sales Team Interactions
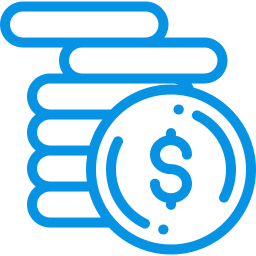
Transactions

Sentiments

Web and Mobile Browsing Activities

Firmographics

Customer Preferences

Etc.
4. Assess the Quality of Your Data: When integrating data from multiple sources at scale, few businesses really understand the underlying data themselves. This data is usually gathered over the course of years, if not decades, and is chock full of issues like typos, irrelevancy, incompleteness, inaccuracy, and lack of standardization. The standardization issue is further magnified when multiple sources come together, because each system may be storing data in a completely different way.
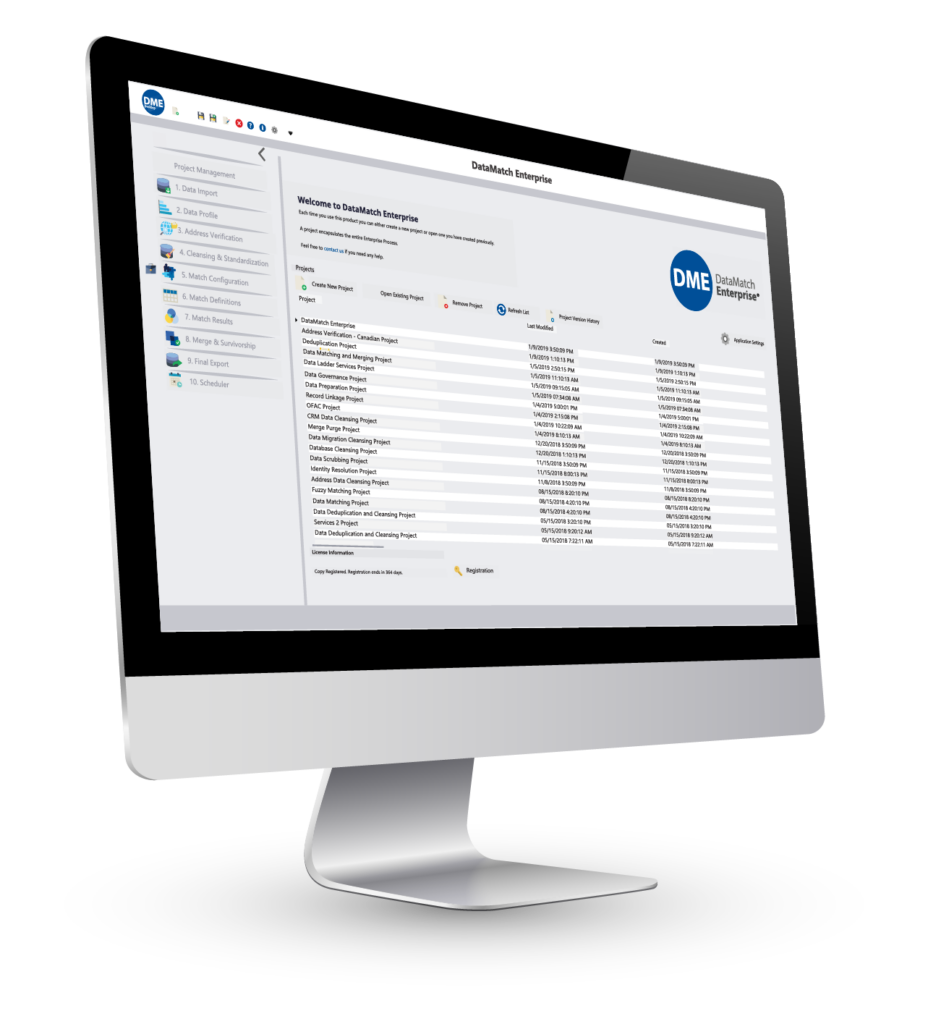
5. Clean & Standardize Your Data: Once you’ve identified issues in your data, it’s time to scrub it clean and standardize it. To clean your data, you can set up business rules that help recognize and fix spelling errors, standardization issues, misfielded data, etc. You should already know which business rules to create if you’ve profiled your data. Keep in mind that this step can be very time consuming and requires considerable attention to detail. For faster, accurate results, data cleansing software is a good choice.
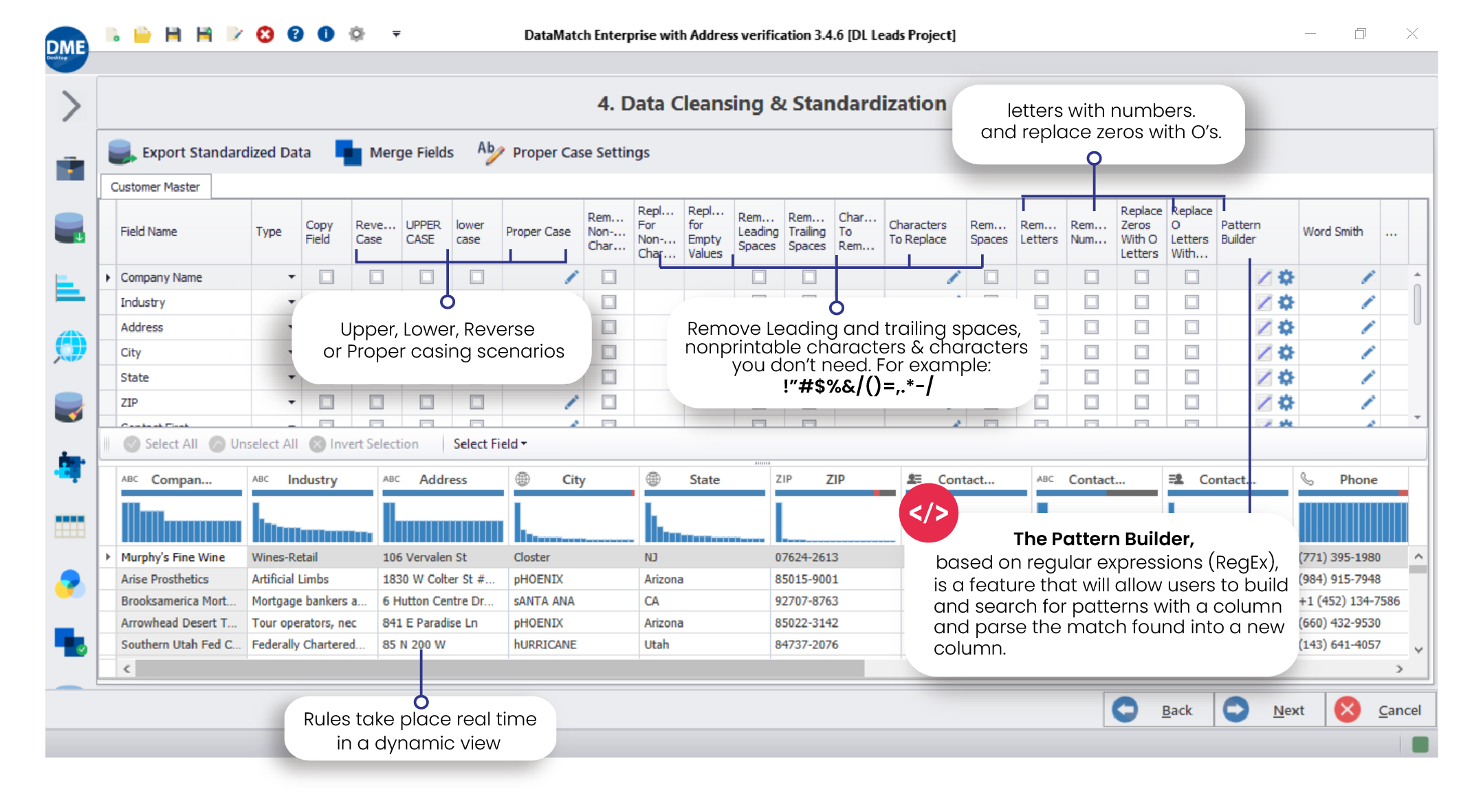
6. Remove Duplicates: Data deduplication removes duplicate items from databases and lists either by matching records manually or using data matching algorithms to automatically detect duplicates. The purpose of deleting duplicate rows/records is to clean the underlying data set to achieve productivity improvements, save on duplicate mailings, and increase customer satisfaction. Manually deleting duplicates can be a time consuming and error prone task, which is why dedupe software is an essential tool for enterprise-wide data quality initiatives.
7. Consolidate Data: Once you’ve deduped your data, it’s time to match your records to get the accurate view of your customer data. At this point, you have already cleansed, standardized and dedupe your data and so the next step of enriching your data with the additional information to formulate the view can be done easily. It’s like joining pieces of a puzzle. You’ve studied the picture, made the plan, sorted the pieces and now it’s just about assembling it.
8. Create a Master Record: As you consolidate this data, you can create a master record. Ideally, a data deduping and cleansing tool should be able to let you create master records while allowing for previous copies to be retained so you can always go back and forth, replacing columns as you deem necessary.
9. Set Up Real Time Data Cleansing: Your data quality is an ongoing challenge. As long as you collect data, it will be inherently flawed. It’s better to set up a real-time data cleansing schedule that ensures your data is free from duplicates and errors as it enters your system.
Make Data Quality a Priority: Don’t take a step back just because you’ve got the data needed for this project. Make data quality a priority. It affects every aspect of your organization. You cannot afford to let data get stagnated. It’s always recommended to run a data quality check after every six months so your data remains updated and usable when needed.